Распознавание цифр с помощью Tesseract OCR и python
Я использую Тессеракт и python для считывания цифр (из счетчика энергии). Все работает хорошо, за исключением числа "1". Тессеракт не может прочитать цифру" 1".
Это фотография, которую я посылаю тессеракту. :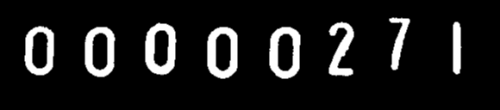
И Тессеракт читает "0000027".
Как я могу сказать Тессеракту, что вертикальный стержень - это "1"?Это моя инициализация Тессеракта:
import tesseract
TESSERACT_LIBRARY_PATH = "C:\Program Files (x86)\Tesseract-OCR"
LANGUAGE = "eng"
CHARACTERS = "0123456789"
FALSE = "0"
TRUE = "1"
def init_ocr():
"""
.. py:function:: init_ocr()
Utilize the Tesseract-OCR library to create an tesseract_ocr that
predicts the numbers to be read off of the meter.
:return: tesseract_ocr Tesseracts OCR API.
:rtype: Class
"""
# Initialize the tesseract_ocr with the english language package.
tesseract_ocr = tesseract.TessBaseAPI()
tesseract_ocr.Init(TESSERACT_LIBRARY_PATH, LANGUAGE,
tesseract.OEM_DEFAULT)
# Limit the characters being seached for to numerics.
tesseract_ocr.SetVariable("tessedit_char_whitelist", CHARACTERS)
# Set the tesseract_ocr to predict for only one character.
tesseract_ocr.SetPageSegMode(tesseract.PSM_AUTO)
# Tesseract's Directed Acyclic Graph.
# Not necessary for number recognition.
tesseract_ocr.SetVariable("load_system_dawg", FALSE)
tesseract_ocr.SetVariable("load_freq_dawg", FALSE)
tesseract_ocr.SetVariable("load_number_dawg", TRUE)
tesseract_ocr.SetVariable("classify_enable_learning", FALSE)
tesseract_ocr.SetVariable("classify_enable_adaptive_matcher", FALSE)
return tesseract_ocr
1 ответ:
Слегка неуместный ответ, хотя и может послужить вашей первоначальной цели.
У меня была аналогичная проблема с Тессерактом, и у меня были очень строгие требования к производительности. Я нашел это простое решение на SO и создал простой распознаватель с OpenCV.Он сводится к поиску ограничивающих прямоугольников (от краев) на очень четком изображении, которое у вас есть, а затем пытается сопоставить найденные объекты с шаблонами. Я верю, что решение в вашем случае будет простым и точным. хотя потребуется немного больше кода, чем у вас есть сейчас.
Я буду следить за этим вопросом, так как будет приятно иметь рабочее решение с Тессерактом.
У меня ограниченное время, но, похоже, это рабочее решение:
import os import cv2 import numpy KNN_SQUARE_SIDE = 50 # Square 50 x 50 px. def resize(cv_image, factor): new_size = tuple(map(lambda x: x * factor, cv_image.shape[::-1])) return cv2.resize(cv_image, new_size) def crop(cv_image, box): x0, y0, x1, y1 = box return cv_image[y0:y1, x0:x1] def draw_box(cv_image, box): x0, y0, x1, y1 = box cv2.rectangle(cv_image, (x0, y0), (x1, y1), (0, 0, 255), 2) def draw_boxes_and_show(cv_image, boxes, title='N'): temp_image = cv2.cvtColor(cv_image, cv2.COLOR_GRAY2RGB) for box in boxes: draw_box(temp_image, box) cv2.imshow(title, temp_image) cv2.waitKey(0) class BaseKnnMatcher(object): distance_threshold = 0 def __init__(self, source_dir): self.model, self.label_map = self.get_model_and_label_map(source_dir) @staticmethod def get_model_and_label_map(source_dir): responses = [] label_map = [] samples = numpy.empty((0, KNN_SQUARE_SIDE * KNN_SQUARE_SIDE), numpy.float32) for label_idx, filename in enumerate(os.listdir(source_dir)): label = filename[:filename.index('.png')] label_map.append(label) responses.append(label_idx) image = cv2.imread(os.path.join(source_dir, filename), 0) suit_image_standard_size = cv2.resize(image, (KNN_SQUARE_SIDE, KNN_SQUARE_SIDE)) sample = suit_image_standard_size.reshape((1, KNN_SQUARE_SIDE * KNN_SQUARE_SIDE)) samples = numpy.append(samples, sample, 0) responses = numpy.array(responses, numpy.float32) responses = responses.reshape((responses.size, 1)) model = cv2.KNearest() model.train(samples, responses) return model, label_map def predict(self, image): image_standard_size = cv2.resize(image, (KNN_SQUARE_SIDE, KNN_SQUARE_SIDE)) image_standard_size = numpy.float32(image_standard_size.reshape((1, KNN_SQUARE_SIDE * KNN_SQUARE_SIDE))) closest_class, results, neigh_resp, distance = self.model.find_nearest(image_standard_size, k=1) if distance[0][0] > self.distance_threshold: return None return self.label_map[int(closest_class)] class DigitKnnMatcher(BaseKnnMatcher): distance_threshold = 10 ** 10 class MeterValueReader(object): def __init__(self): self.digit_knn_matcher = DigitKnnMatcher(source_dir='templates') @classmethod def get_symbol_boxes(cls, cv_image): ret, thresh = cv2.threshold(cv_image.copy(), 150, 255, cv2.THRESH_BINARY) contours, hierarchy = cv2.findContours(thresh, cv2.RETR_EXTERNAL, cv2.CHAIN_APPROX_SIMPLE) symbol_boxes = [] for contour in contours: x, y, width, height = cv2.boundingRect(contour) # You can test here for box size, though not required in your example: # if cls.is_size_of_digit(width, height): # symbol_boxes.append((x, y, x+width, y+height)) symbol_boxes.append((x, y, x+width, y+height)) return symbol_boxes def get_value(self, meter_cv2_image): symbol_boxes = self.get_symbol_boxes(meter_cv2_image) symbol_boxes.sort() # x is first in tuple symbols = [] for box in symbol_boxes: symbol = self.digit_knn_matcher.predict(crop(meter_cv2_image, box)) symbols.append(symbol) return symbols if __name__ == '__main__': # If you want to see how boxes detection works, uncomment these: # img_bw = cv2.imread(os.path.join('original.png'), 0) # boxes = MeterValueReader.get_symbol_boxes(img_bw) # draw_boxes_and_show(img_bw, boxes) # Uncomment to generate templates from image # import random # TEMPLATE_DIR = 'templates' # img_bw = cv2.imread(os.path.join('original.png'), 0) # boxes = MeterValueReader.get_symbol_boxes(img_bw) # for box in boxes: # # You need to label templates manually after extraction # cv2.imwrite(os.path.join(TEMPLATE_DIR, '%s.png' % random.randint(0, 1000)), crop(img_bw, box)) img_bw = cv2.imread(os.path.join('original.png'), 0) vr = MeterValueReader() print vr.get_value(img_bw)